主要な大学、著名研究者、および50万人を超えるMLエンジニア
からの信頼を得ています
からの信頼を得ています
研究および教育向けW&B
Weights & Biasesの引用方法
W&Bを使用した研究の成功事例などがある場合、詳細内容をお聞かせください。右側にある情報を使って弊社を引き合いに出すことができますが、弊社では、貴社の作業についてぜひ直接お聞きしたいと思います。電子メール(research@wandb.com)でご連絡ください。
W&B引用方法を確認 →
@misc{wandb,
title = {Experiment Tracking with Weights and Biases},
year = {2020},
note = {Software available from wandb.com},
url={https://www.wandb.com/},
author = {Biewald, Lukas},
}
title = {Experiment Tracking with Weights and Biases},
year = {2020},
note = {Software available from wandb.com},
url={https://www.wandb.com/},
author = {Biewald, Lukas},
}
一般的なあらゆるフレームワークおよび数千のMLレポジトリと統合
Weights & Biasesはさまざまなツールで適切に動作します。PyTorch、KerasおよびJAXから、MLランドスケープ全体の特定分野のレポジトリまで、弊社の製品は問題なく統合できます。弊社のドキュメントで、最も一般的な統合を(およびどのように動作するか)確認してください。

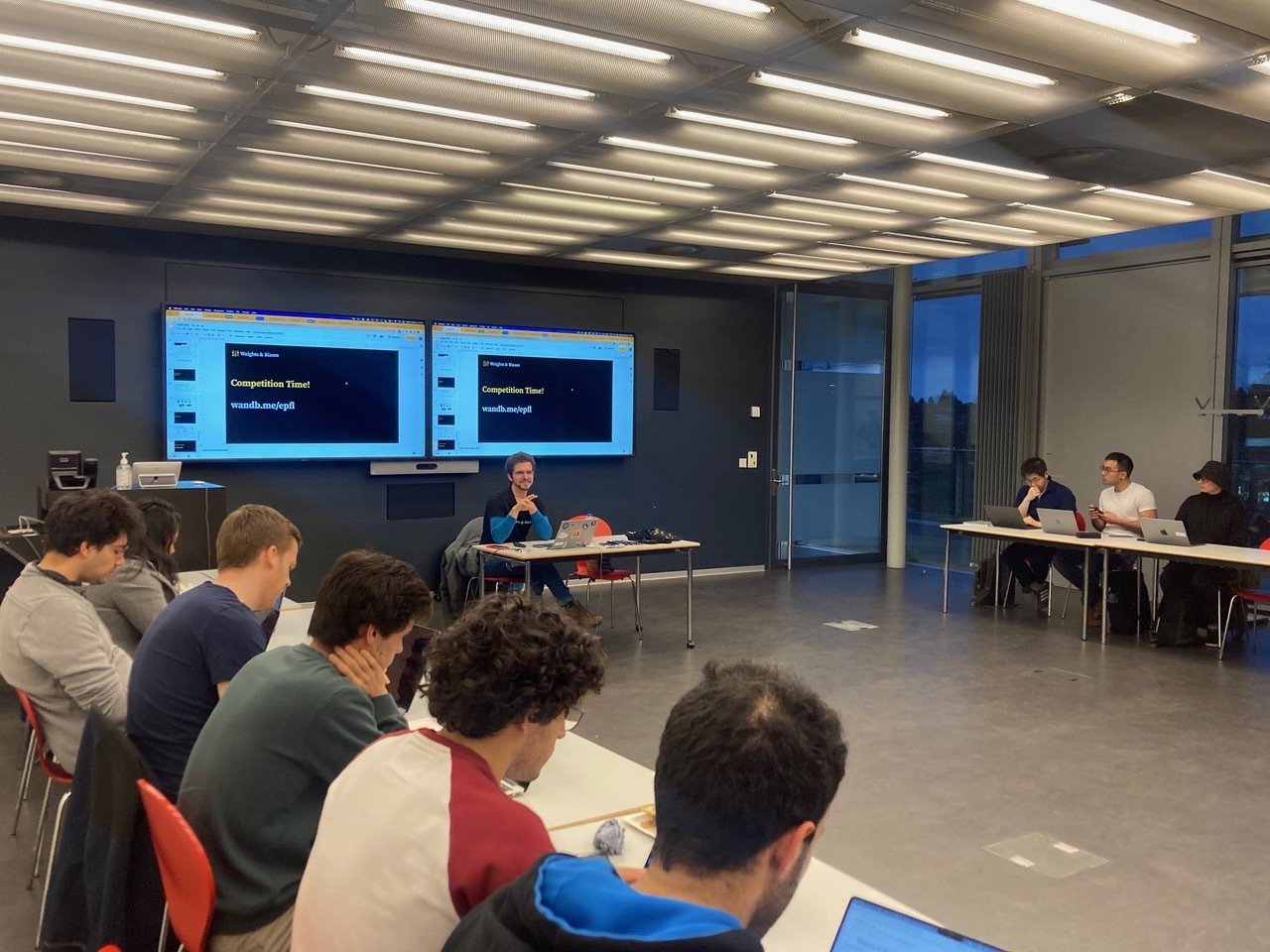
教育者、授業助手、学生向けリソース
あなたと生徒が、教室、実験室、または学生が運営する団体でWeights & Biasesを使って、共同で再現可能な機械学習とディープラーニングを実現するのを支援するための入門的コンテンツが含まれています。
無料リソースを見る →
専門家からMLOpsについて学ぶ
このW&Bの多様な無料コースで、モデル開発を加速および拡大し、生産性を高め、再現性とモデルの履歴を確実にし、そして何よりも、より優れたモデルを素早く学習する方法を発見してください。
無料で登録 →

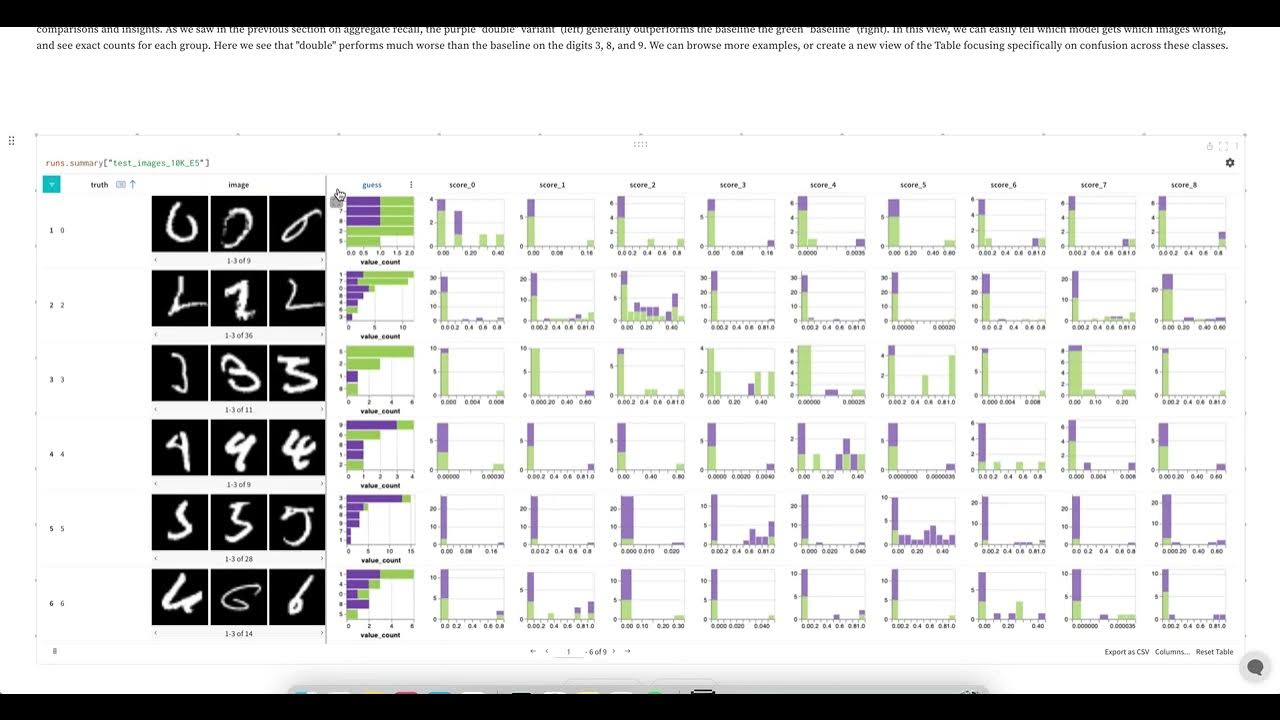
リアルタイムでチームとコラボレーション
Weights & Biasesはコラボレーション向けに作られています。ありとあらゆる実験を単一システムに記録することで、研究チームはすべてのデータセットとモデルバージョン、git commit、および最新の実験に対するアクセスを共有することができます。
チームの作業方法を学ぶ →
Weights & Biases MLOps プラットフォーム
簡単なインストール、高い利便性
ML実験をすべて1か所でトラッキング、比較、可視化。学術関係者や研究者は、弊社の一式の機能すべてにアクセスでき、コード5行だけで開始できます。
LIVE NOTEBOOKを試す
# Flexible integration for any Python script
import wandb
# 1. Start a W&B run
wandb.init(project='gpt4')
config = wandb.config
config.learning_rate = 0.01
# 2. Save model inputs and hyperparameters
# Model training here
# 3. Log metrics over time to visualize performance
wandb.log({"loss": loss})
import wandb
# 1. Start a W&B run
wandb.init(project='gpt3')
# 2. Save model inputs and hyperparameters
config = wandb.config
config.learning_rate = 0.01
# Model training here
# 3. Log metrics over time to visualize performance
with tf.Session() as sess:
# ...
wandb.tensorflow.log(tf.summary.merge_all())
import wandb
# 1. Start a new run
wandb.init(project="gpt-3")
# 2. Save model inputs and hyperparameters
config = wandb.config
config.learning_rate = 0.01
# 3. Log gradients and model parameters
wandb.watch(model)
for batch_idx, (data, target) in
enumerate(train_loader):
if batch_idx % args.log_interval == 0:
# 4. Log metrics to visualize performance
wandb.log({"loss": loss})
import wandb
from wandb.keras import WandbCallback
# 1. Start a new run
wandb.init(project="gpt-3")
# 2. Save model inputs and hyperparameters
config = wandb.config
config.learning_rate = 0.01
... Define a model
# 3. Log layer dimensions and metrics over time
model.fit(X_train, y_train, validation_data=(X_test, y_test),
callbacks=[WandbCallback()])
import wandb
wandb.init(project="visualize-sklearn")
# Model training here
# Log classifier visualizations
wandb.sklearn.plot_classifier(clf, X_train, X_test, y_train, y_test, y_pred, y_probas, labels, model_name='SVC', feature_names=None)
# Log regression visualizations
wandb.sklearn.plot_regressor(reg, X_train, X_test, y_train, y_test, model_name='Ridge')
# Log clustering visualizations
wandb.sklearn.plot_clusterer(kmeans, X_train, cluster_labels, labels=None, model_name='KMeans')
# 1. Import wandb and login
import wandb
wandb.login()
wandb.login()
# 2. Define which wandb project to log to and name your run
wandb.init(project="gpt-3", run_name='gpt-3-base-high-lr')
# 3. Add wandb in your Hugging Face `TrainingArguments`
args = TrainingArguments(... , report_to='wandb')
# 4. W&B logging will begin automatically when your start training your Trainer
trainer = Trainer(... , args=args)
trainer.train()
trainer.train()
import wandb
# 1. Start a new run
wandb.init(project="visualize-models", name="xgboost")
# 2. Add the callback
bst = xgboost.train(param, xg_train, num_round, watchlist, callbacks=[wandb.xgboost.wandb_callback()])
# Get predictions
pred = bst.predict(xg_test)